5.4. Orthogonal projection#
An interesting problem is to find the point of a subset that is located at the smallest possible distance from a given point. The solution to this particular type of constrained optimization problem is called orthogonal projection. It generalizes the notion of projection from linear algebra, and it is a building block of many constrained optimization algorithms.
1 - Definition. The orthogonal projection of a point \({\bf u}\in\mathbb{R}^N\) onto a subset \(\mathcal{C}\subset \mathbb{R}^N\) is formally defined as
In general, there may exist several orthogonal projections of a point onto a set, provided that the latter is nonempty and closed. The above \(\operatorname{Argmin}\) (with uppercase ‘A’) denotes the set of all the points of \(\mathcal{C}\) at the same smallest distance from \({\bf u}\), and the inclusion symbol (\(\in\)) is there to remind that the orthogonal projection \(\mathcal{P}_{\mathcal{C}}({\bf u})\) can be any of those points.
2 - Convexity. The orthogonal projection is unique when the set \(\mathcal{C}\) is convex. In this case, the projection can be precisely defined as the unique point of \(\mathcal{C}\) at the smallest distance from \({\bf u}\) (note the \(\operatorname{argmin}\) is now with lowercase ‘a’):
3 - Geometrical optimality. In any case, the necessary optimality condition states that the direction from the projection \(\mathcal{P}_{\mathcal{C}}({\bf u})\) to the original point \({\bf u}\) is orthogonal to the set \(\mathcal{C}\) at \(\mathcal{P}_{\mathcal{C}}({\bf u})\):
This explains why it is called “orthogonal projection”. Some examples are illustrated below.
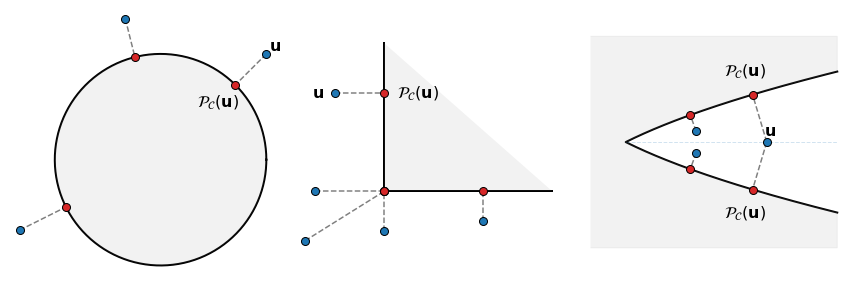
4 - Properties. Open the sections below to learn more about the orthogonal projection and its various properties.
Idempotence
By looking at the definition, it is immediately evident that any point of \(\mathcal{C}\) is the projection of itself:
The projection is thus an idempotent operation, because \(\mathcal{P}_{\mathcal{C}}(\mathcal{P}_{\mathcal{C}}({\bf u})) = \mathcal{P}_{\mathcal{C}}({\bf u})\) for any point \({\bf u} \in \mathbb{R}^N\).
Cartesian product
A constraint of \(\mathbb{R}^{N}\) may be defined as the cartesian product of several sets \(\mathcal{C}_k\subset \mathbb{R}^{N_k}\) of smaller dimensions such that \(N=N_1+\dots+N_K\), namely
A vector of \(\mathcal{C}_{\rm prod}\) is decomposed in blocks, and each block belongs to the corresponding \(\mathcal{C}_k\):
Then, the projection onto \(\mathcal{C}_{\rm prod}\) concatenates the projections onto each \(\mathcal{C}_k\):
Intersection
A constraint of \(\mathbb{R}^{N}\) may be defined as the intersection of several sets \(\mathcal{C}_k\subset \mathbb{R}^{N}\) of equal dimension:
A vector of \(\mathcal{C}_{\rm cross}\) belongs to all \(\mathcal{C}_k\) simultaneously:
The projection onto \(\mathcal{C}_{\rm cross}\) cannot be written as a simple combination of projections onto each \(\mathcal{C}_k\):
Shift
A constraint of \(\mathbb{R}^{N}\) may be defined as the points of a set \(\mathcal{C}\subset \mathbb{R}^{N}\) shifted by a vector \({\bf r}\in\mathbb{R}^{N}\):
The projection onto \(\mathcal{C}_{\rm shift}\) is related to the projection onto \(\mathcal{C}\) by a simple shift:
Linear transform
A constraint of \(\mathbb{R}^{N}\) may be defined as the points of a set \(\mathcal{C}\subset \mathbb{R}^{K}\) after a multiplication by \(A\in\mathbb{R}^{K\times N}\):
If \(A\) is a \(\nu\)-frame with \(\nu>0\), the projection onto \(\mathcal{C}_{\rm linear}\) is related to the projection onto \(\mathcal{C}\) as follows:
Note that NO formula exists in the general case when \(A A^\top \neq \nu I\).
Spectral sets
When the optimization is taken with respect to symmetric (square) matrices, a constraint of \(\mathbb{R}^{N\times N}\) may be defined as the matrices with eigenvalues belonging to a set \(\mathcal{C}\subset \mathbb{R}^{N}\):
where \(\lambda_W\in\mathbb{R}^{N}\) denotes the vector of eigenvalues of \(W\). The projection onto \(\mathcal{C}_{\rm eig}\) is related to the projection onto \(\mathcal{C}\) as follows:
where \(U=Q_U\,{\rm diag}(\lambda_U)\,Q_U^\top\) is a spectral decomposition of \(U\). When the optimization is taken with respect to rectangular matrices, the singular value decomposition replaces the spectral decomposition.